Artificial intelligence (AI) is evolving at an astonishing pace, shaping industries, economies, and daily life in ways we could have never imagined. Yet, with this remarkable progress comes a pressing challenge: uncertainty. As you dive deeper into the world of AI, you may find yourself grappling with questions about its capabilities, limitations, and the risks it poses. This uncertainty can feel overwhelming, but it’s also a powerful force driving new research, innovative solutions, and a deeper understanding of AI’s potential.
In this article, we will explore the sources of uncertainty in AI, how researchers are working to manage it, and the future outlook for a more transparent and reliable AI landscape. By the end, you’ll have a clearer understanding of how uncertainty in AI affects you, whether you’re a consumer, professional, or AI enthusiast.
Table of Contents
Understanding Uncertainty in AI: A Growing Concern
AI systems are not infallible. In fact, one of the greatest challenges facing AI today is its inherent uncertainty. But what exactly is uncertainty in AI? At its core, it refers to the unpredictable nature of AI’s behavior and decisions. Whether it’s the outcome of a machine learning algorithm or the response of an AI-powered chatbot, uncertainty is a constant factor that researchers are working to minimize.(uncertainty in ai)
Why Does Uncertainty in AI Matter to You?
Uncertainty in AI can have profound consequences. In industries like healthcare, autonomous driving, and finance, the stakes are high, and even a slight mistake can lead to costly errors or catastrophic consequences. For instance, a medical AI tool that misinterprets a diagnosis could jeopardize a patient’s health. Similarly, an autonomous vehicle that misjudges a pedestrian’s movement could lead to an accident. You may not realize it, but AI is already playing a role in your daily life, and its uncertainty affects you in ways you might not fully grasp.(uncertainty in ai)
Key Sources of Uncertainty in AI Research
To better understand how uncertainty creeps into AI systems, let’s break it down into key sources that researchers and developers are actively addressing.
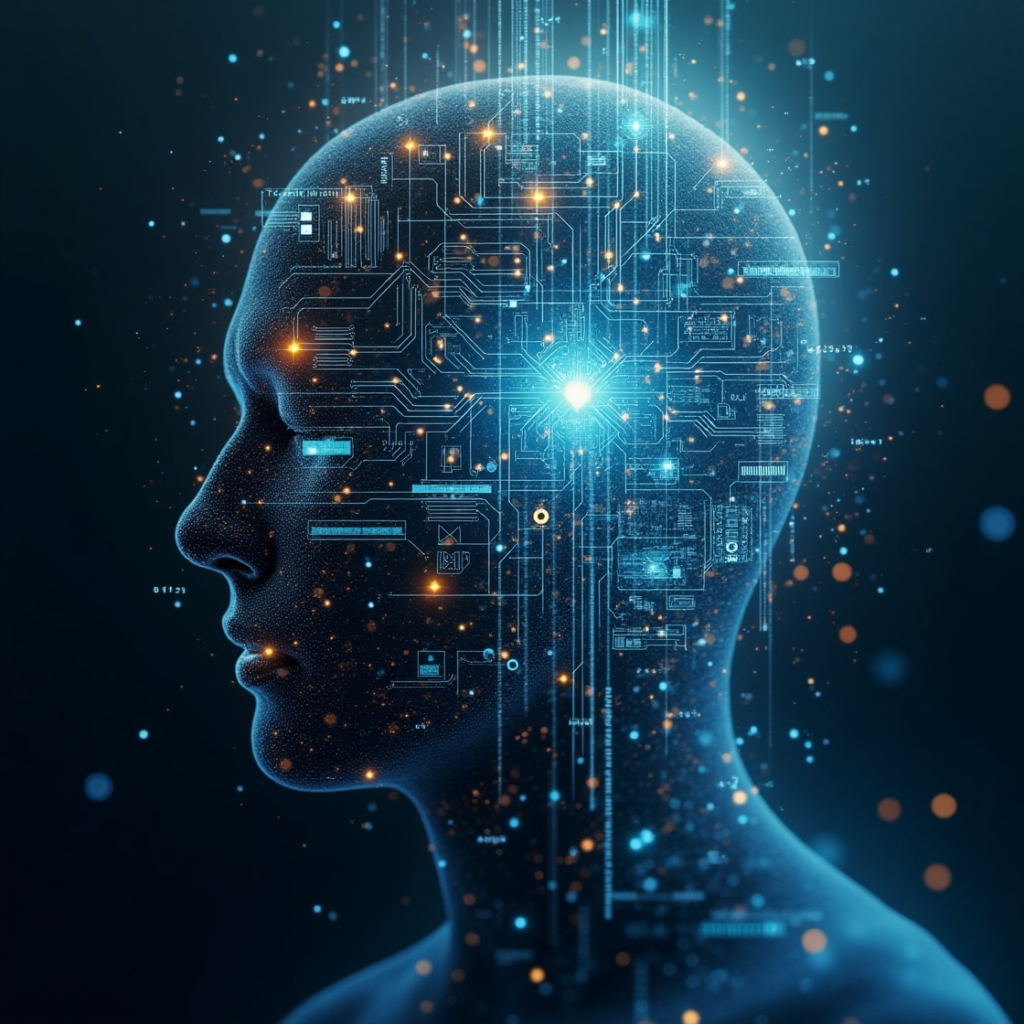
Data-Related Uncertainty
What Is It?
AI systems rely heavily on data to function. The data fed into an AI model shapes its ability to learn and make decisions. However, when the data is flawed—whether it’s incomplete, biased, or noisy—the model’s predictions and decisions become uncertain. This is especially concerning in fields like healthcare, where inaccurate data could lead to dangerous consequences.
How It Affects You
Imagine you’re using an AI-based health monitoring app that helps track your vitals and provides suggestions for better health. If the data used to train the app is biased or flawed, the advice you receive could be misleading, which affects your wellbeing.
Algorithmic Uncertainty
What Is It?
AI algorithms are designed to process and analyze data to make decisions or predictions. However, the process is far from perfect. Machine learning models, particularly deep learning algorithms, can behave unpredictably due to their complexity. Even slight changes in input data can lead to significant variations in output, creating uncertainty in the AI’s results.(uncertainty in ai)
How It Affects You
If you’ve ever used a recommendation system (like Netflix or Amazon) and found that it doesn’t always recommend what you expect, it’s a perfect example of algorithmic uncertainty. The model may have had difficulty predicting your preferences accurately, leading to an unsatisfactory experience.(uncertainty in ai)
Model Uncertainty
What Is It?
Model uncertainty refers to the challenges AI faces when applying learned knowledge to real-world scenarios. Models can behave inconsistently, especially when they encounter situations that differ from the data used during training. The unpredictability of these models is a key source of uncertainty in AI development.
How It Affects You
In industries like autonomous driving, model uncertainty can be especially dangerous. For example, a self-driving car’s AI might perform flawlessly under normal circumstances, but when faced with an unexpected road situation, its decision-making process could be flawed, leading to accidents or delays.
Research Insights: Tackling Uncertainty in AI Development
Researchers are working tirelessly to address these sources of uncertainty. In fact, some groundbreaking advancements have already begun to mitigate these issues. Let’s take a look at some of the most promising strategies.
Advancements in AI Explainability
What Is Explainable AI (XAI)?
AI explainability refers to the ability of AI systems to provide transparent and understandable reasons behind their decisions. By making AI systems more interpretable, researchers aim to reduce uncertainty and enhance trust among users.
Why It Matters to You
If you’ve ever felt uneasy using AI in decision-making processes (such as a loan application or job selection), explainable AI offers a solution. When AI models can explain why they made a particular decision, it builds confidence and helps mitigate uncertainty. For example, an AI-powered job screening tool that explains why a candidate was chosen or rejected can prevent feelings of unfair treatment and increase trust in the system.
Uncertainty Quantification Techniques
What Are They?
Quantifying uncertainty is a technique used to measure how confident an AI model is in its predictions. Techniques like Bayesian methods and Monte Carlo simulations help researchers estimate the uncertainty in model outputs and adjust accordingly.
Why It Matters to You
Imagine a financial advisor AI that helps you make investment decisions. By quantifying the uncertainty in its predictions, it can provide you with more accurate risk assessments. This can help you make more informed choices and avoid major losses in the stock market.
AI Robustness and Generalization
What Is It?
AI robustness refers to the ability of an AI system to perform well even when faced with unexpected or noisy data. Generalization refers to the ability of an AI model to apply its knowledge to new, unseen situations. Both of these aspects are critical in reducing uncertainty.
Why It Matters to You
AI systems that are both robust and generalizable will perform more reliably in the real world. If you’re using AI for something critical, such as autonomous vehicles or medical diagnostics, you want to be sure that the system can handle a wide range of scenarios without breaking down. Researchers are working to make AI systems more adaptable, which is crucial for minimizing uncertainty.
Ethical Considerations and the Impact of Uncertainty in AI
As AI continues to evolve, ethical concerns surrounding its uncertainty become more pressing. It’s essential to address these concerns to ensure that AI serves humanity in a fair and responsible manner.
Bias and Fairness
What Is It?
Bias in AI refers to the phenomenon where AI systems produce skewed or unfair outcomes, often due to biased training data. This can lead to discrimination against certain groups of people, creating ethical dilemmas and amplifying uncertainty.
How It Affects You
You’ve probably heard of instances where AI systems have been criticized for racial or gender bias, such as facial recognition technology misidentifying people of color more frequently than white individuals. This kind of bias can perpetuate inequality and cause harm, making it crucial to address the uncertainty associated with biased AI systems.
Trust and Accountability in AI
What Is It?
AI systems must be accountable for their actions.In the event an AI system errs, who bears the responsibility? By integrating accountability mechanisms into AI development, researchers aim to ensure that AI remains reliable and trustworthy.
Why It Matters to You
Imagine a situation where an AI system makes a mistake that causes harm or financial loss. Who would you hold responsible? Researchers are working to create systems that ensure AI can be held accountable, reducing the uncertainty surrounding its use in high-stakes situations.
Case Studies: Real-World Examples of AI Uncertainty
To better understand how uncertainty in AI plays out in real-world applications, let’s explore some compelling case studies.
Autonomous Vehicles
The Challenge of Uncertainty
Autonomous vehicles rely heavily on AI to navigate and make decisions on the road. However, the unpredictable nature of real-world environments—such as weather conditions, road construction, or erratic drivers—creates significant uncertainty for self-driving cars.
How It’s Being Addressed
Researchers are working on improving the robustness of AI models, making autonomous vehicles more capable of handling unexpected scenarios. This includes better sensor technology, real-time data analysis, and enhanced decision-making algorithms.
Healthcare AI
The Challenge of Uncertainty
AI-powered tools are increasingly being used to assist in diagnosing diseases and recommending treatments. However, the uncertainty of medical data and the complexity of human health make it difficult for AI systems to provide 100% accurate predictions.
How It’s Being Addressed
To address this uncertainty, researchers are focusing on improving the quality of medical data and integrating human expertise with AI-powered diagnostic tools. By combining the power of AI with human intuition, healthcare professionals can make more reliable decisions.
Finance and Trading
The Challenge of Uncertainty
AI is widely used in the finance industry to predict market trends and make investment decisions. However, financial markets are highly volatile, and even the most advanced AI systems can struggle with predicting market fluctuations.
How It’s Being Addressed
AI systems are being improved with better data models, uncertainty quantification, and risk assessment techniques. These advancements help investors and financial institutions make more informed decisions despite the inherent uncertainty in the market.
The Future of AI and Overcoming Uncertainty
As AI continues to evolve, researchers are discovering new ways to reduce uncertainty and improve its reliability. While there’s still much work to be done, the future of AI holds great promise.
The Path Forward
Emerging Trends
The future of AI involves creating systems that are not only more robust and transparent but also better at handling uncertainty. Researchers are working on techniques like reinforcement learning, transfer learning, and hybrid AI models to improve AI’s adaptability and decision-making.
AI and Human Collaboration
AI is not meant to replace humans; it’s meant to enhance human capabilities. By collaborating with AI, humans can make better, more informed decisions, especially when uncertainty is a factor.
Conclusion: Embracing the Unknown – A Brighter Future for AI
AI’s uncertainty may feel daunting at times, but it’s also a catalyst for innovation. By understanding the sources of uncertainty and supporting the ongoing research to address them, we can help shape a future where AI systems are not only smarter but also more trustworthy and reliable. As AI continues to evolve, you can be a part of this exciting journey—navigating the unknown with curiosity, confidence, and a commitment to ethical responsibility.
Call to Action
Are you prepared to delve further into the realm of AI? Stay informed by subscribing to our newsletter and joining the conversation on how AI is transforming industries, tackling uncertainty, and paving the way for the future.